We're just in the wrap-up for Slider-0.80-incubating; A windows VM nearby is busy downloading the source .zip file and verifying that it builds & tests on windows.
Before people feel sorry for me consider this: given a choice between windows C++ code and debugging Kerberos, I think I'd rather bring up MSDN in a copy of IE6 while playing edit-and-continue games in Visual Studio than try and debug the obscure error messages you get with kerberos on Java. Not only are they obscure, my latest Java 7 update event changed the text of the error which means "now we've upgraded the JVM you don't have the encryption settings to use kerberos".
Which is a shame, because I have to make sure everything works with kerberos.
Anyway: build works and the tests are running —which keeps me happy. If all goes to plan, Slider 0.80-incubating will be there for download within a week.
Some of the new features include
- SLIDER-780: Ability to deploy docker packages
- SLIDER-663 zero-package cluster definition. (It has a different name, but it essentially means "no need to build a redistributable zip file for each application). While the zip-based distribution is essential for things you want to share, for things you are developing or using yourself, this is lighter weight.
- SLIDER-733 Ability to install a package on top of another one. This addresses "the coprocessor problem": how to add a new HBase coprocessor JAR without rebuilding everything. And, with SLIDER-633, you can define that new package easily,
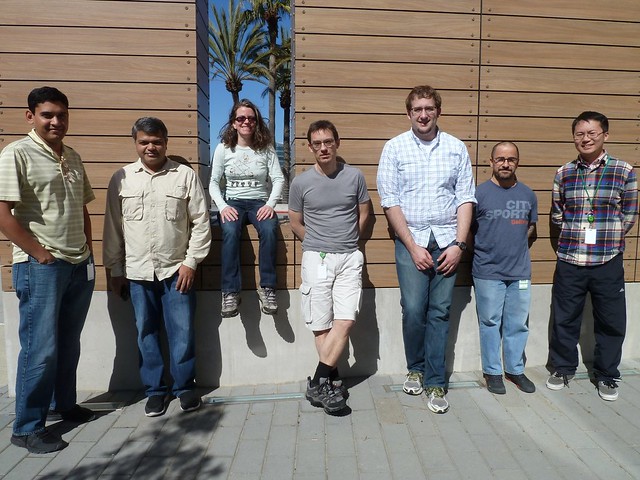
As anyone who knows me will realise: that's because my Hortonworks colleagues are really great people who know more about computing than I ever have or will and are better at applying that knowledge than myself —someone who uses "test driven development" as a way of hiding his inability to get anything to work right first time.
And yet these people still allow me to work with them —showing there still things that they consider something I'm still up to handling.
What have I been up to? Placement, specifically SLIDER-611: Über-JIRA - placement phase 2
Right from the outset, Hadoop HDFS and MR have been built on some assumptions about failure, availability and bandwidth
- Disks will fail: deal with it by using replication over 2h replacement part support contracts and RAID-array rebuilding
- Servers will fail: app developers have to plan for that.
- Some servers are just unreliable; apps may work this out without even needing to be told. Example: stragglers during a map identifies servers whose disks may be in trouble.
- If an application fails a few times it's unreliable and should be killed.
- The placement/scheduling of work is primarily an optimisation to conserve bandwidth. That is: you place work for proximity to the desired data.
- If the desired placement cannot be obtained, placement elsewhere is usually acceptable.
- It's better to have work come up fast somewhere near the data than wait for minutes for it to potentially come up on the actual node requested.
- If work is placed on a different machine, the cost to other apps (i.e. the opportunity cost) is acceptable.
- It's OK if two containers get allocated to the same machines ("affinity is not considered harmful")
- The actions of one app running on a node are isolated from the others (e.g. CPU throttling and vmem limiting is sufficient to limit resource conflict).
- Work has a finite duration, and containers know when they are finished. Nothing else needs to make that decision for them.
But what about long lived services, such as HBase and Kafka?
HBase uses short-circuit reads for maximum performance working with local data. Although region servers don't have to be co-located with the data, until there's a full Hbase compaction, most of the data for an RS is likely to be remote. (for each Block b, P(data-local) = f(nodes/3), (roughly; the 3-replica-2-rack policy complicates the equation as blocks are not spread completely randomly).
Therefore: restarting on the wrong node can slow down that region server's performance for an extended period of time.
HBase is often used low-latency apps; if other things are running there then it can impact performance. That is, you'd rather not have all the CPU + storage capacity taken up by lower priority work if that work tangibly impacted network and disk.
If you've configured the HBase rest and thrift servers with hard coded ports, they are at risk of conflicting for port numbers with other services.
Kafka? If you bring up a kafka instance on another node, all its local data is lost and it has to rebuild it from the last snapshot+stream. This is expensive; cost O(elapsed-time-since-snapshot * event arrival rate). Once rebuilt, performance recovers.
Kafka loves anti-affinity in placement; reduces the no. of instances that die on a node failure, and impact on the system until the rebuild is complete.
Both these apps then are things you want to deploy under YARN, but they have very different scheduling and placement requirements.
Long-lived services in general
- May be willing to wait for an extended period to come up on the same server as before. That is, even if a server has crashed and is rebooting, or is down for a quick hardware fix —its better to wait before giving up.
- But they can recover, and ultimately giving up is desireable.
- Unreliability is not a simple metric of failures, it's failures in a recent time period that matters. That holds for the entire application, as well as distributed components.
- Can fail in interesting ways.
With slider's goal "support long-lived services in YARN without making you rewrite them", we see that difference and get to address it.
YARN Labels
A key feature is in Hadoop 2.6: labels. (YARN-796). Admins can give nodes labels; give queues shared/exclusive access to sets of labelled nodes, give users those rights.
Slider picks this up by allowing you to assign different components to different labels. The region servers in a production HBase cluster could all be given the property yarn.label=production;
We're using labels to isolate bits of the cluster for performance. They all share HDFS, so there is some cross-contamination, but IO-heavy analytics work can be kept off the nodes. We'd really like HDFS prioritisation for even better isolation, such as giving shortcut-reads priority over TCP traffic. Future work.
You can also split up a heterogenous cluster, with GPU or SSD labels, more RAM nodes etc. Even if an app isn't coded for label awareness, you can (in the Capacity Scheduler) get different queues to manage labels, so grant different users access to the nodes.
One thing that's interesting to consider is, in an EC2 cluster, labelling nodes as full vs spot-priced. You could place some work on spot-priced nodes, others on full. Not only does this give better guarantees of existence, if HDFS is only running on the full nodes, different performance characteristics. I'd be interested to know of any experiences here.
Placement Escalation.
SLIDER-799 AM to decide when to relax placement policy from specific host to rack/cluster
This kept me busy in March; a fun piece of code.
As mentioned earlier, YARN schedulers like to schedule work fast, even if non-local. An am can ask for "do-not-relax" placement, but then there's no relaxation even if a node never comes back.
What we've done is taken the choice about when to relax out of YARN's hands and into the AMs. By doing so, you can specify a time delay in minutes to hours, rather than relying on YARN to find a space and having it back off in a few tens of seconds at most.
This is easier to summarise than go through the details. For the curious, the logic to pick a location is in RoleHistory; escalation in OutstandingRequestTracker. Note that code is all part of our Model; we don't let that directly interact with YARN, which is something for what is controller's task. The model builds up a list of Operations which are then processed afterwards. This really helps testing: we can test the entire model through a mock YARN cluster, taking the actions and simulating their outcome, then add failure events, restarts, etc. Fast tests for fast dev cycles.
Node reliability tracking
We've had this for a while, not with explicit blacklisting but basic greylisting, building up a list of nodes we don't trust and never asking for them explicitly. What's changed is the sophistication of listing and how we react to it.
- We differentiate failure types; Node failure counters discard those which are node-independent (example: container memory limits exceeded), and those which are simply pre-emption events. (SLIDER-856).
- Similarly, component role failure counters don't count node failures or pre-emption in the reliability statistics of a role.
- The counters of role & node failures used for deciding, respectively if an app is failing or a node is unreliable , are reset on a regular, schedule basis (a few hours, tunable).
- If we don't trust a node, we don't ask for containers on it, even if is the last place where it ran. (Exception: if you declare that a component placement policy is "strict". It's always asked for again, and there is no escalation).
Where next?
Absolutely key is anti-affinity. I don't see YARN-1042 coming soon —but that's OK. Now we do our own escalation, we can integrate that with anti affinity.
How? ask for a container at a time, blacklisting all those nodes where we've already got an instance. Ramp-up time will be slower, especially taking in to account that escalation may result in container allocations taking minutes before a dead/overloaded node is given up on.
Maybe it could be something like
- inital request: blacklist all but those we last ran on.
- escalation: relax to all but: nodes those with outstanding requests or allocated containers -or considered too unreliable.
- do this in parallel, discarding allocations which assign >1 instance to the same node.
- If, after a certain time, nodes are still unallocated, maybe consider relaxing restriction (as usual: configurable policy and timeouts per role)
I need to think about this a bit more to see if it would work, estimate ramp-up times, etc.
Anything else?
Look at Über-JIRA : placement phase 3 for some ideas.
Otherwise, SLIDER-109, Detect and report application liveness. Agents could report in URLs they've built for liveness probes, either they check themselves or the AM hits them all on a schedule (with monitor threads designed to detect the probes themselves hanging). All the code for this is from the Hadoop 1 HA monitor work I did in 2012; it's checked in waiting to be wired up. All we need it someone to do the wiring. Which is where the fact that slider is an OSS project comes in to play.
- All of the implemented features listed here are available to anyone who wants to download and run Slider.
- All the new ideas are there for someone to implement. Join the team! Check out the source, enhance it, write those simulation tests, submit patches!
And it's really interesting. I could get distracted putting in time on this. Indeed, SLIDER-856 kept me busy two weekends ago to the extent that I got told off for irresponsible role modelling (parental, not slider codebase). Apparently spending a weekend in front of a monitor is a bad example for a teenage boy. But the placement problem is not just something I find interesting. Read the Borg paper and notice how they call out placement across failure domains and availability zones. They've hit the same problems. Add it to slider and collect real world data and you've got insight into scheduling and placing cluster workloads that even Google would be curious about.
So: come and play.
No comments:
Post a Comment
Comments are usually moderated -sorry.